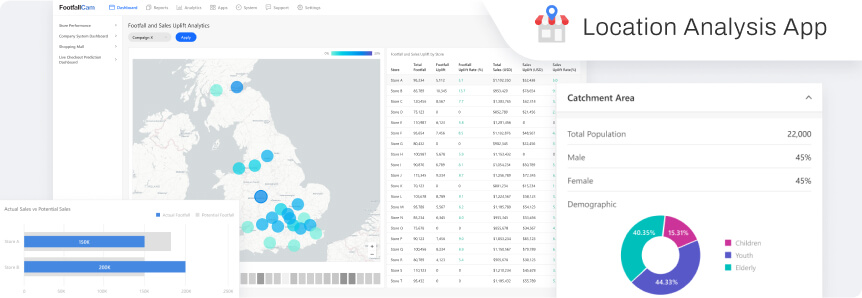
A leading retailer faced the annual challenge of identifying and addressing non-performing stores. Despite overall business success, the company had to remain vigilant, addressing issues related to poor operations, data accuracy, and potential job losses at underperforming locations. Closing a store is a complex, costly decision that impacts employees, customers, and the brand. To make the best decisions, the retailer sought to leverage AI-powered insights to discern when the store location was the primary issue and ensure closure was the last resort.
The retailer adopted an AI model specifically designed for in-depth location analysis. This data-driven approach offered a clear, objective view of each store’s potential, enabling the real estate director to navigate this difficult task with greater confidence and reduced uncertainty. The AI model became an essential part of the executive team, delivering actionable insights and saving considerable time in the decision-making process.
Pain Points
1. Operational and Data Challenges: Poor operations and inaccurate data led to the misidentification of underperforming stores, impacting profitability and operational efficiency.
2. Risk of Workforce Reduction: Closing a store directly affects employees, so making accurate, well-supported decisions is crucial to minimise unnecessary job losses.
3. Costly and Impactful Decisions: Store closure requires significant capital and resource allocation, making it essential that closures are only executed when location performance cannot be improved by other means.
The AI model provided the following critical insights and analysis capabilities:
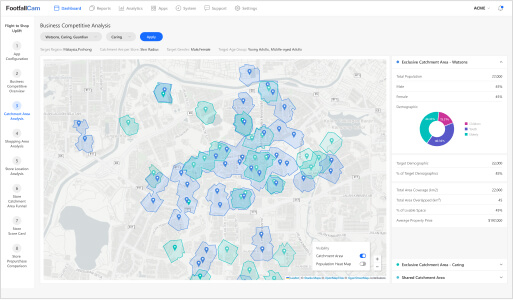
Location Potential Evaluation
The AI assessed historical data, foot traffic, and local demographics, enabling the retailer to determine whether low performance was due to the location itself or other factors. By analysing the store’s potential based on its surroundings and competitor proximity, the AI identified which stores could realistically become profitable with the right adjustments versus those where closure was the best option.
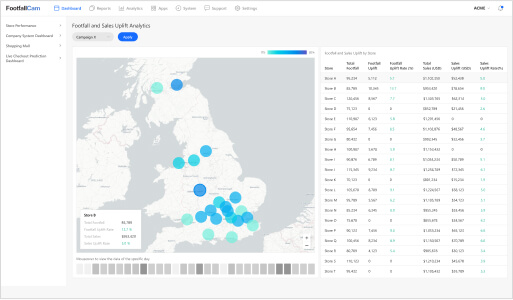
Operational Performance Analysis
The AI model analysed store performance against similar locations across the portfolio, highlighting disparities that pointed to operational inefficiencies or other issues, like inventory or staffing, rather than location alone. This feature helped differentiate between stores that needed operational improvements and those where closure was necessary.
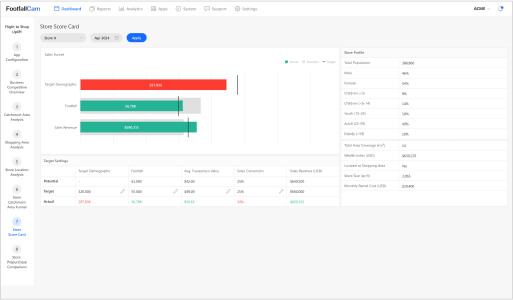
Predictive Data-Driven Decision-Making
The AI provided predictive insights into future store performance by examining trends in footfall, customer spending, and nearby developments, allowing the real estate director to make pre-emptive adjustments. By simulating different scenarios, such as changes in staffing, layout, or product offerings, the AI offered insights into possible outcomes and supported informed, data-driven decisions.
Strategic and Efficient Store Closures
With the Store Closure Optimisation Report, the real estate director was able to:
1. Implement Strategic Closures: Based on predictive data and operational insights, the retailer closed only locations where location was the confirmed primary issue, ensuring that closures were a last resort.
2. Preserve Jobs Where Possible: By distinguishing operational challenges from true location-based issues, the retailer reduced the number of closures, preserving jobs at stores with untapped potential.
3. Improve Overall Store Portfolio: Through AI-driven insights, the retailer was able to optimise its store portfolio, eliminating high-risk locations while bolstering investment in profitable stores, enhancing overall profitability.
The use of an AI model for location analysis empowered the retailer to navigate the complex process of identifying and closing non-performing stores.
The AI tool provided crucial insights that saved time and reduced uncertainty, transforming the executive decision-making process. This data-driven approach not only allowed the real estate director to make well-supported closure decisions but also helped mitigate the impact on employees and the business. By leveraging technology, the retailer demonstrated that AI can be a vital asset in strategic business optimisation, ensuring that every decision aligns with long-term profitability and operational resilience.This case study highlights the power of data-driven insights in modern retail management, showing how AI can play a transformative role in maintaining a healthy, profitable store portfolio.
Know more about Location Analysis App